There Must Be a Better Way: Moving Beyond Labels in a Data-Rich World
Most of what I write on pauldobinson.com is grounded in the safe terrain of technology, sport, and business. But sometimes, something personal lingers long enough to demand reflection. This post is one of those. It’s different. And I hesitated to write it.
Because it’s about fairness. About identity. About systems built with good intent, which—over time—can quietly start to feel like they’re missing the mark.
This post isn’t about outrage. It’s about curiosity. It’s about standing at the crossroads of four countries I’ve lived in or spent time in—South Africa, the UK, Australia, and the U.S.—and asking:
In a world of data, intelligence, and nuance, are we still building systems that exclude people… just differently?
From Personal to Systemic
In South Africa, I heard from young white South Africans—smart, driven, modest backgrounds—who couldn’t get into university, or were overlooked for jobs. Not because they lacked merit, but because of policy. Black Economic Empowerment (BEE) was designed to address decades of oppression. And it has. But like many systems, it’s created new edges—ones where ability sometimes takes a backseat to background.
In Australia, I once received feedback after being passed over for a promotion. My capability wasn’t in question. I just didn’t bring the “right” diversity. My gender was cited directly.
And then there’s my late father—a man with a career of leadership and military service behind him. In his 50s, he couldn’t find work. He no longer fit the profile that companies were looking for: middle-aged, white, straight, male.
These aren’t sob stories. I’ve done well. I know I sit in a place of privilege. But the point isn’t about me.
It’s about a deeper pattern I see across borders: systems that elevate people based on what they represent, not who they are.
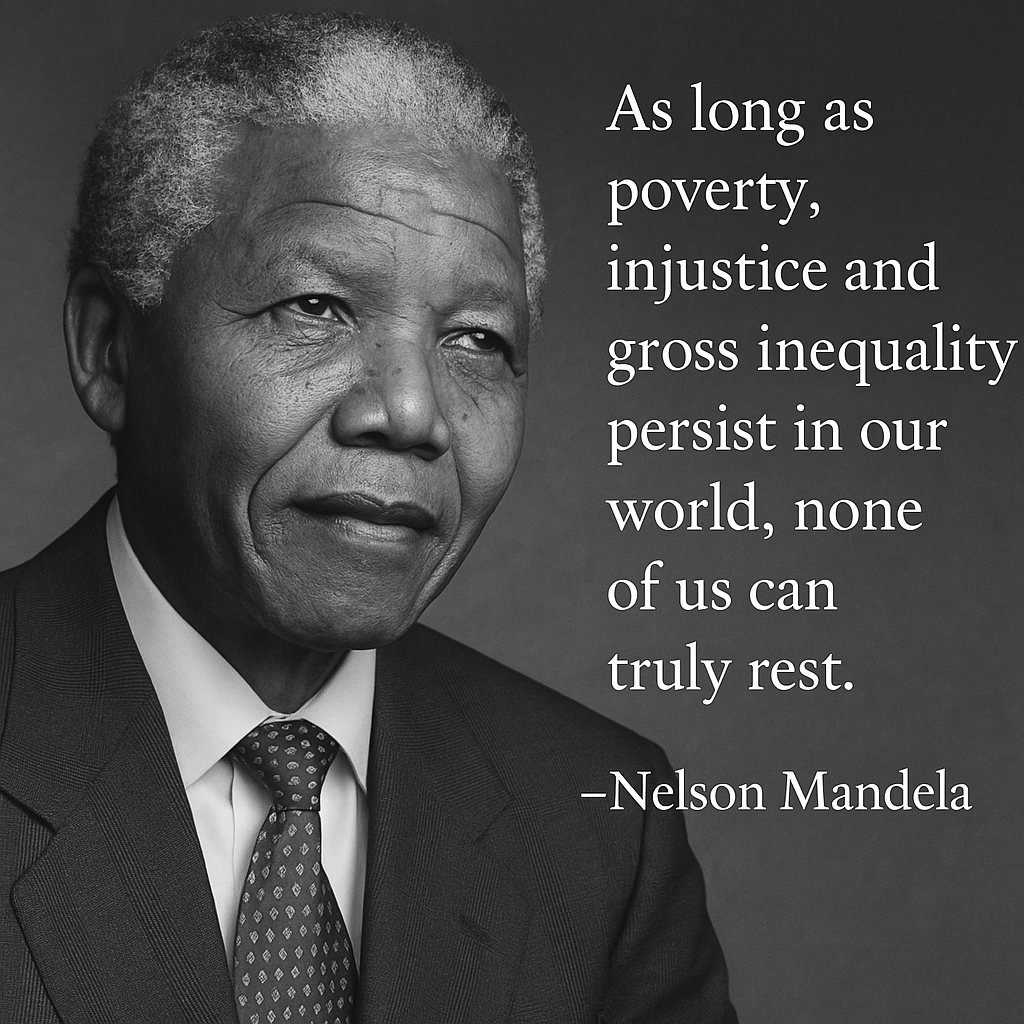
Global Fractures, Global Questions
These aren’t just local stories—they’re part of a broader pattern.
In the United States, the DEI conversation has exploded. In 2025, President Trump revoked Executive Order 11246, removing affirmative action from federal contracts. Elon Musk, now heading the “Department of Government Efficiency,” helped dismantle DEI programs across government. Some celebrated it. Others protested. Foreign allies, like France, pushed back against U.S. pressure to export these ideas globally.
In South Africa, youth unemployment is over 45.5%, and white emigration is on the rise. Some leave because they feel unwelcome. Others because they no longer see opportunity—regardless of their talent or values.
In the UK, the Civil Service has quietly implemented a Contextual Recruitment Tool, which takes socioeconomic background into account—not just grades. The results? A doubling of success rates for underrepresented applicants, without lowering standards.
In Australia, I’ve seen the rise of women in leadership—many of them exceptional. But I’ve also seen cases where advocacy for gender equity has led to visibility and platforms that fast-tracked people beyond what their track record might otherwise have warranted.
We rarely say it out loud. But in whisper-level conversations, we ask: is this the right way?
Beyond Labels
We still lean heavily on labels: race, gender, sexuality, age.
These categories help us measure systemic inequality. But when we use them as shortcuts for opportunity, we risk replacing one kind of bias with another.
We need to evolve.
We have the tools.
We have the data.
And we have no excuse.
What Better Could Look Like
Some models point toward that better way:
- The UK’s CRT values hardship, not just grades. It adds context instead of taking identity at face value.
- Salesforce uses real-time tracking to expose gaps in pay, promotion velocity, and leadership diversity. It doesn’t force diversity—but it sees clearly where systems are breaking down.
- Pymetrics and similar platforms assess candidates based on traits, not resumes. They look for potential, not polish.
And yet, even good intentions can misfire.
In 2024, Google’s AI image tool overcorrected by depicting historical figures in ways that ignored context. The result? Confusion, backlash, and eroded trust. Inclusion doesn’t work if it flattens history—or people.
Lessons from AI Fluency
While studying AI Fluency at the University of Sydney, I was impressed by how seriously the issue of bias was taken. We explored how AI can perpetuate discrimination if trained on flawed data. We looked at how to fix it: algorithm audits, inclusive training sets, human oversight.
The message was simple:
AI is not neutral. But it can be powerful—if designed with care, curiosity, and ethics.
It was a reminder that labels are only one data point. If we build systems to actually see people—to understand their stories, their skills, their growth—then maybe we can start making decisions based on individuals, not archetypes.
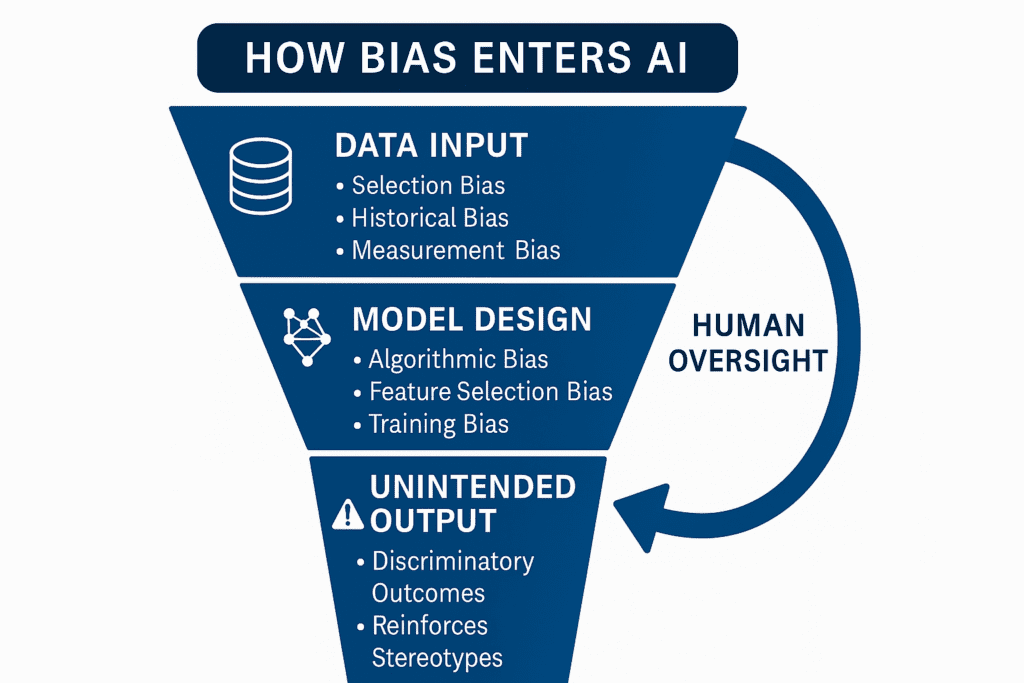
So… What Are We Really Solving?
Equity isn’t a checkbox. It’s a craft.
And it requires intersectionality—recognising that race, gender, class, and background all intertwine.
But it also requires us to stop treating identity as merit.
That’s the uncomfortable part. It’s possible to champion inclusion and question how opportunity is handed out. It’s possible to believe in fairness while asking if some of the systems we’ve built are just new versions of gatekeeping.
This post doesn’t give you answers. It gives you a pause.
To reflect.
To consider.
To ask, like I’ve been asking:
In a world with this much data, this much intelligence, and this much lived experience…
There must be a better way.
Further Reading & Resources
- 🔍 University of Sydney – AI Fluency Microcredential
A great foundation in understanding how AI can support or harm equity—and how to do it right.
Explore AI Fluency - 📊 Salesforce Equality Initiatives
Real-time insights into how one of the world’s most influential tech companies tracks fairness in practice.
Salesforce Equality - 🇬🇧 UK Civil Service – Contextual Recruitment Tool
Learn how data-driven hiring improves representation and outcomes.
Government CRT Guide - 📉 The Verge – Google’s AI Overcorrection Case
A cautionary tale in algorithmic inclusion without context.
Read the Article - 📖 Harvard Business Review – AI Bias and What to Do About It
Practical insights on bias mitigation for organisations using machine learning.
HBR: AI Bias
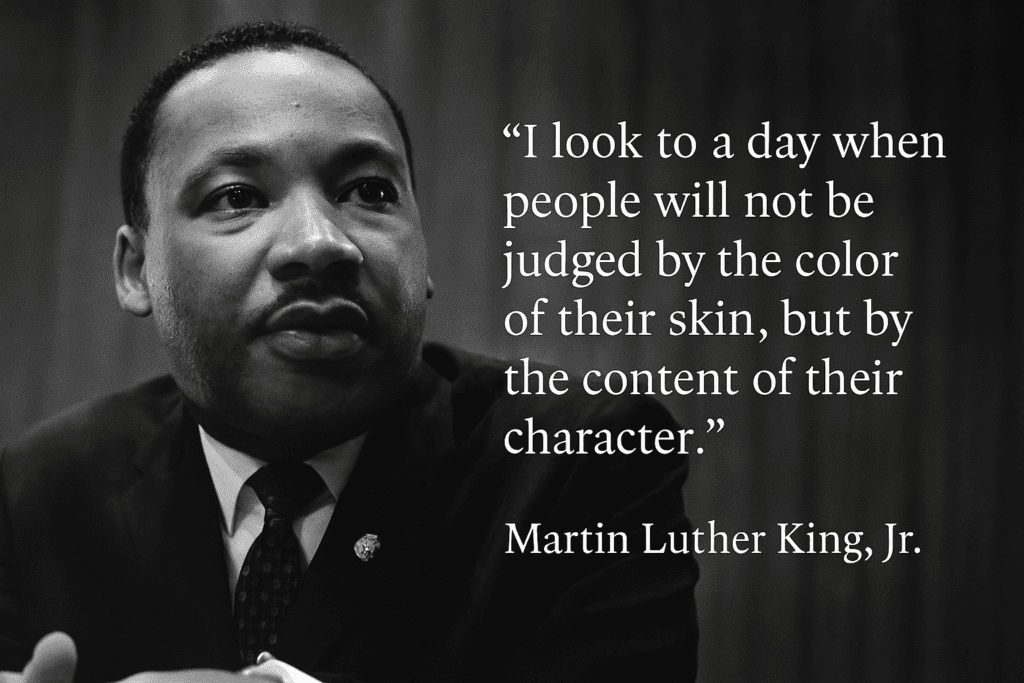